Visual Analytics for Time-dependent Data
- Admin
- 2 de nov. de 2019
- 2 min de leitura
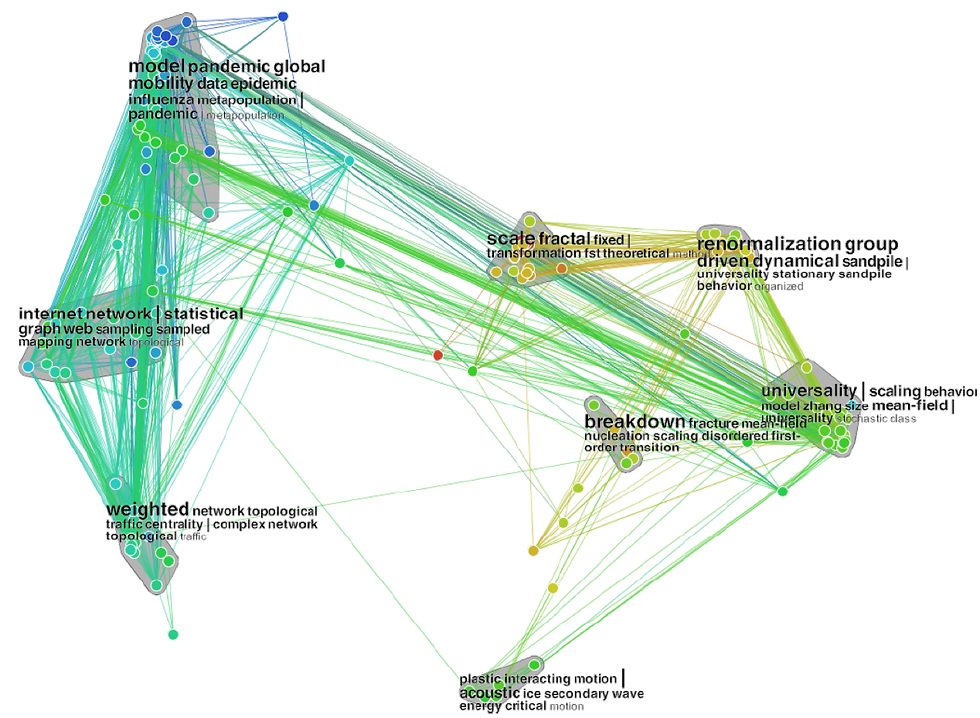
Consider the tasks of summarizing a patent collection over the course of time to reveal the advances in a knowledge domain; assessing the evolution of an athlete’s performance according to different parameters; analyzing an outbreak disease with the intent to understand the mechanisms of an epidemic propagation and potentially how to control it; or monitoring the environment to evaluate its evolution or the impact of specific variables on an ecosystem.
The key concept underlying these applications is capturing the evolution of a phenomenon over time. Although some visualization techniques can be used for the analysis of time-dependent aspects, most of them are only suitable for unidimensional time-series data. With data that cannot be directly modeled as time-series, such as unstructured document collections and dynamic graphs, there is an acute need for new techniques to support the discovery and representation of trends and patterns that evolve over time. The proposed research will develop new approaches for the analysis of temporal aspects of non-time-series datasets, aiming to devise tools and techniques to support the understanding and interpretation of time-dependent patterns on large volumes of data.
Appropriately handling the time dependency imposes several challenges for user-centered analytics environments, especially the cognitive overload imposed on users on the analysis of multiple concurrent or dependent patterns. The proposed research will investigate new visual metaphors and techniques, taking advantage of machine-automated approaches to defining a multilevel pattern-centered exploratory process that allows users to navigate and explore time-varying data at different levels of abstraction and refinement. We intend to deliver solutions not only for persistent data but also for streaming data, that is, data that is continuously produced and need to be processed as received.
With industrial and academic collaborations, we will test the proposed methods in three different domains: unstructured time-dependent data; time-varying graphs; and trajectory data. Examples of applications that will benefit from the advances proposed here include sports performance analysis, analysis of trends in text collections, and environmental monitoring, potentially impacting and improving the daily routines of both expert and casual users.
Comments